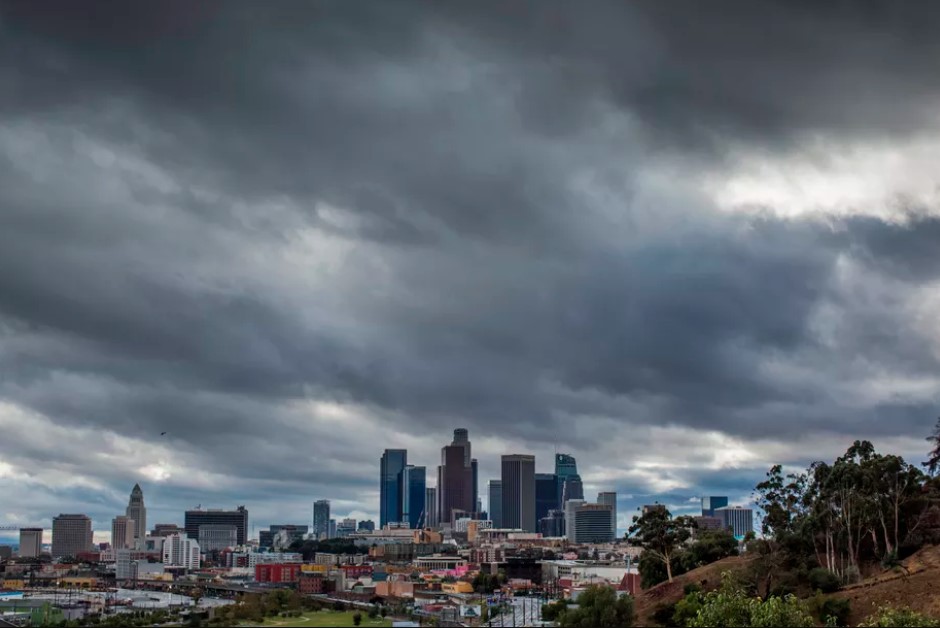
Weather forecasting is notoriously difficult, but in recent years experts have suggested that machine learning could better help sort the sunshine from the sleet. Google is the latest firm to get involved, and in a blog post this week shared new research that it says enables “nearly instantaneous” weather forecasts.
The work is in the early stages and has yet to be integrated into any commercial systems, but early results look promising. In the non-peer-reviewed paper, Google’s researchers describe how they were able to generate accurate rainfall predictions up to six hours ahead of time at a 1km resolution from just “minutes” of calculation.
That’s a big improvement over existing techniques, which can take hours to generate forecasts, although they do so over longer time periods and generate more complex data.
Speedy predictions, say the researchers, will be “an essential tool needed for effective adaptation to climate change, particularly for extreme weather.” In a world increasingly dominated by unpredictable weather patterns, they say, short-term forecasts will be crucial for “crisis management, and the reduction of losses to life and property.”
/cdn.vox-cdn.com/uploads/chorus_asset/file/19599987/WeatherPatterns.gif)
The biggest advantage Google’s approach offers over traditional forecasting techniques is speed. The company’s researchers compared their work to two existing methods: optical flow (OF) predictions, which look at the motion of phenomenon like clouds, and simulation forecasting, which creates detailed physics-based simulations of weather systems.
The problem with these older methods — particularly the physics-based simulation — is that they’re incredibly computationally intensive. Simulations made by US federal agencies for weather forecasting, for example, have to process up to 100 terabytes of data from weather stations every day and take hours to run on expensive supercomputers.
“If it takes 6 hours to compute a forecast, that allows only 3-4 runs per day and resulting in forecasts based on 6+ hour old data, which limits our knowledge of what is happening right now,” wrote Google software engineer Jason Hickey in a blog post.
Google’s methods, by comparison, produce results in minutes because they don’t try to model complex weather systems, but instead make predictions about simple radar data as a proxy for rainfall.
The company’s researchers trained their AI model on historical radar data collected between 2017 and 2019 in the contiguous US by the National Oceanic and Atmospheric Administration (NOAA). They say their forecasts were as good as or better than three existing methods making predictions from the same data, though their model was outperformed when attempting to make forecasts more than six hours ahead of time.
This seems to be the sweet spot for machine learning in weather forecasts right now: making speedy, short-term predictions, while leaving longer forecasts to more powerful models. NOAA’s weather models, for example, create forecasts up to 10 days in advance.
While we’ve not yet seen the full effects of AI on weather forecasting, plenty of other companies are also investigating this same area, including IBM and Monsanto. And, as Google’s researchers point out, such forecasting techniques are only going to become more important in our daily lives as we feel the effects of climate change.